CEO Tech Commentary: UAV navigation in high-altitude or GNSS-denied environments
An interview with Aliaksei Stratsilatau, CEO of UAVOS
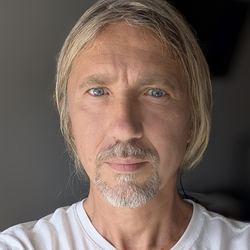
Aliaksei Stratsilatau, CEO of UAVOS, shares expert insights into the solutions the company uses to enable unmanned aircraft to navigate in high-altitude or GNSS-denied environments.
What technologies do you employ to maintain accurate navigation in high-altitude or GNSS-denied environments?
There are several solutions we use to allow an unmanned aircraft to navigate by estimating its global position.
One of these is air data dead-reckoning. It actually involves using airspeed measurements to extrapolate the craft’s trajectory, thereby estimating its global position. This works in conjunction with an inertial measurement unit (IMU). IMUs are usually based on MEMS (Microelectromechanical systems) technology, and are not as precise or as expensive as some of the big laser gyros and complex navigation units.
We combine MEMS technology with dead-reckoning based on air data, enabling navigation in GNSS-denied environments. However, this approach introduces some measurement errors due to unknown atmospheric conditions.
The autopilot can estimate wind only when GPS is available, so without it, we lack information on wind direction, speed and changes over time. As a result, errors are tied to these wind parameters – meaning that strong winds can push the craft off-course, and the airspeed sensors won’t detect this drift.
The second technology involves radio modems. For long-range communication with a UAV, we typically use tracking antenna systems on the ground. These systems employ a directional antenna that continuously points toward the aircraft by automatically tracking it. They rely on the aircraft’s global position to maintain accurate alignment.
But we can also use radio modems that receive information on signal strength to adjust the antenna position, and capture and follow the aircraft. This is because in GNSS- or GPS-denied environments – or even those with bad signals – we have no global positioning information, and can only track the aircraft using the strength of the datalink signal. By using the signal strength parameter, we can determine the bearing of the aircraft from the ground and estimate its global position through triangulation.
Some radio modems, particularly those we use and develop, also enable us to measure the distance between radios. By using this distance measurement as feedback, as well as the bearing of the aircraft, we can estimate its global positioning, and send global position fixes straight to the aircraft, eliminating the error [of drift].
The third technology is one we use when an aircraft is within our line-of-sight from the ground. Intended as a short fix that uses new corrected measurements – thus eliminating IMU and dead-reckoning errors – we send an adjustment from ground control, telling the UAV where it is. We’ve combined these three methods together to solve the problem in the past, and it works pretty well.
Of course, everything needs further development to improve its autonomous operation. Everything I mentioned is connected to the ground, and everything implicitly assumes you have a ground control station and a human operator etc., so if we were to move to autonomous operation today, everything would become more complicated, and this is where AI technologies, especially AI imaging, come into the game.
In autonomous navigation, AI imaging often involves capturing images of the ground with a downward-facing camera. An onboard processor then compares this ground imagery with a preloaded map, such as Google Earth data, to estimate the UAV’s location. All computations are performed onboard to reduce the need for high-bandwidth data transmission to the ground and to avoid latency.
This [estimation] can be done in one of two ways, either you upload some reference points with images that AI can easily recognise (some people in the past have used QR Codes or unique buildings on the ground, for example), or you can train your onboard AI by making previous flights in the area with GPS enabled, and collect all the necessary data for the neural network that way. Then you can continue the same operation in the same area, in GPS-denied situations. Additionally, there’s a technology known as "optical flow," which estimates velocity vectors by analysing consecutive video frames. This method helps further reduce IMU drift errors by providing more accurate movement data.
We also used the computer vision in our drone navigation systems. The UAVOS autopilot system leveraged computer vision and AI to navigate the UAV in GNSS-denied environments. The most important part is the hardware that actually enables the onboard AI processing.The equipment we used was based on Nvidia’s AI processing modules, which are the leading hardware products used for AI processing.
The onboard computer vision-based alternative navigation module with deep learning algorithms provided the UAVOS avionics system with the geospatial coordinates. Computer vision enabled accurate, resilient navigation during both day and night, offering safe take-off and landing independent of the UAV. This method for acquiring and processing enabled the drone to "see" and interpret its environment, allowing it to navigate autonomously without needing GNSS.
One of these is air data dead-reckoning. It actually involves using airspeed measurements to extrapolate the craft’s trajectory, thereby estimating its global position. This works in conjunction with an inertial measurement unit (IMU). IMUs are usually based on MEMS (Microelectromechanical systems) technology, and are not as precise or as expensive as some of the big laser gyros and complex navigation units.
We combine MEMS technology with dead-reckoning based on air data, enabling navigation in GNSS-denied environments. However, this approach introduces some measurement errors due to unknown atmospheric conditions.
The autopilot can estimate wind only when GPS is available, so without it, we lack information on wind direction, speed and changes over time. As a result, errors are tied to these wind parameters – meaning that strong winds can push the craft off-course, and the airspeed sensors won’t detect this drift.
The second technology involves radio modems. For long-range communication with a UAV, we typically use tracking antenna systems on the ground. These systems employ a directional antenna that continuously points toward the aircraft by automatically tracking it. They rely on the aircraft’s global position to maintain accurate alignment.
But we can also use radio modems that receive information on signal strength to adjust the antenna position, and capture and follow the aircraft. This is because in GNSS- or GPS-denied environments – or even those with bad signals – we have no global positioning information, and can only track the aircraft using the strength of the datalink signal. By using the signal strength parameter, we can determine the bearing of the aircraft from the ground and estimate its global position through triangulation.
Some radio modems, particularly those we use and develop, also enable us to measure the distance between radios. By using this distance measurement as feedback, as well as the bearing of the aircraft, we can estimate its global positioning, and send global position fixes straight to the aircraft, eliminating the error [of drift].
The third technology is one we use when an aircraft is within our line-of-sight from the ground. Intended as a short fix that uses new corrected measurements – thus eliminating IMU and dead-reckoning errors – we send an adjustment from ground control, telling the UAV where it is. We’ve combined these three methods together to solve the problem in the past, and it works pretty well.
Of course, everything needs further development to improve its autonomous operation. Everything I mentioned is connected to the ground, and everything implicitly assumes you have a ground control station and a human operator etc., so if we were to move to autonomous operation today, everything would become more complicated, and this is where AI technologies, especially AI imaging, come into the game.
In autonomous navigation, AI imaging often involves capturing images of the ground with a downward-facing camera. An onboard processor then compares this ground imagery with a preloaded map, such as Google Earth data, to estimate the UAV’s location. All computations are performed onboard to reduce the need for high-bandwidth data transmission to the ground and to avoid latency.
This [estimation] can be done in one of two ways, either you upload some reference points with images that AI can easily recognise (some people in the past have used QR Codes or unique buildings on the ground, for example), or you can train your onboard AI by making previous flights in the area with GPS enabled, and collect all the necessary data for the neural network that way. Then you can continue the same operation in the same area, in GPS-denied situations. Additionally, there’s a technology known as "optical flow," which estimates velocity vectors by analysing consecutive video frames. This method helps further reduce IMU drift errors by providing more accurate movement data.
We also used the computer vision in our drone navigation systems. The UAVOS autopilot system leveraged computer vision and AI to navigate the UAV in GNSS-denied environments. The most important part is the hardware that actually enables the onboard AI processing.The equipment we used was based on Nvidia’s AI processing modules, which are the leading hardware products used for AI processing.
The onboard computer vision-based alternative navigation module with deep learning algorithms provided the UAVOS avionics system with the geospatial coordinates. Computer vision enabled accurate, resilient navigation during both day and night, offering safe take-off and landing independent of the UAV. This method for acquiring and processing enabled the drone to "see" and interpret its environment, allowing it to navigate autonomously without needing GNSS.
March, 2025